OpenAI's big announcement: Why enterprises should pay attention

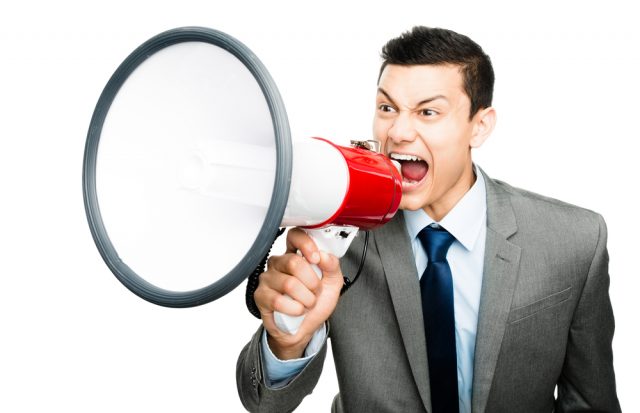
OpenAI held its first dev day conference last week, and announcements there made huge waves in technology and startup circles. But it’s enterprises that should be paying attention, and here’s why:
OpenAI made significant improvements to ChatGPT -- ones that address critical flaws that made it unsuitable for enterprise use cases because the results were inaccurate, non-credible and untrustworthy. What’s changed is that OpenAI has integrated retrieval augmented generation (RAG) into ChatGPT.
Initially developed by Meta, RAG is an AI technique that combines the power of retrieval-based models (access to real-time data + domain-specific data) with generative models (natural language responses).
Without RAG, generative AI tools like ChatGPT that use general purpose large language models (LLMs):
- Can’t access real-time information.
- Can’t access domain-specific or custom datasets.
- Frequently fabricate responses (hallucinations!).
Enterprise AI use cases are knowledge-intensive ones that involve large volumes of domain-specific data -- and have a high bar for accuracy, credibility and transparency.
That’s why OpenAI’s adoption of RAG makes sense. They’ve closed some big holes with this move. And it’s why companies like Google, Amazon, Microsoft and many startups have been building generative AI solutions using RAG.
So, does this mean ChatGPT is now ready for the enterprise? The answer, of course, is it depends!
ChatGPT now, by default, browses Bing (which means it can access real-time information) and can cite its sources (making it less prone to hallucinations). Users can also upload custom and domain-specific datasets.
Those exploring a RAG-based tool like ChatGPT that uses a general purpose LLM should know that they need to invest in making it work for their enterprise use case.
Recognize that ChatGPT is browsing the entire internet, which means it will access and cite both credible and non-accurate sources. Users will have to invest in further prompt engineering to circumvent this or source, curate and provide domain-specific or custom data themselves.
In addition, users will need to train retrieval models to tailor document ranking based on user context and relevance. They also must fine tune LLMs to understand the input language style as well as to respond in the appropriate output tone and terminology to be usable by the enterprise.
The alternative is domain-specific RAG-based solutions that are emerging to address common enterprise use cases, and these can often be leveraged out of the box with little or no customization.
The new general rule for enterprise AI technology selection:
- Explore general purpose RAG-based tools -- and the effort required to customize them -- for highly bespoke use cases that existing domain-specific solutions don’t address.
- Explore domain-specific RAG-based solutions purpose-built to address any specific use cases at hand -- where those solutions are available.
The good news is that enterprise organizations have more AI options than ever before, and innovation in both underlying technology and enterprise-grade solutions is moving at breakneck pace.
Photo Credit: Bevan Goldswain/Shutterstock
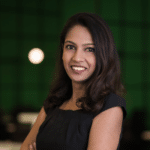
Chandini Jain is the founder and CEO of Auquan, AI innovator transforming the world’s unstructured data into actionable intelligence for financial services customers. Prior to founding Auquan, Jain spent 10 years in global finance, working as a trader at Optiver and Deutsche Bank. She is a recognized expert and speaker in the field of using AI for investment and ESG risk management. Jain holds a master's degree in mechanical engineering/computational science from the University of Illinois at Urbana-Champaign and a B.Tech from IIT Kanpur. For more information on Auquan, visit www.auquan.com/, and follow the company @auquan_ and on LinkedIn.