The role of AI in securing identity [Q&A]

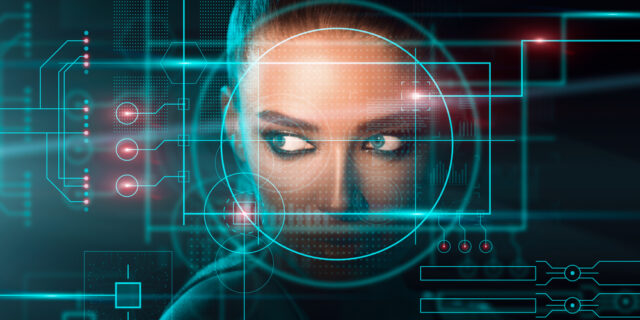
Identities are probably the biggest attack surface for organizations in today's world as employees rely more on systems and apps to do their jobs.
Mapping identity and access data from the large, disparate, and often disconnected, mix of on-premise and cloud systems that enterprises use is a major challenge.
We sat down with Craig Davies, CISO of Gathid, to discuss why identity has become such a massive attack surface and the role that AI is playing in helping secure identity across organizations.
BN: How has the cybersecurity landscape shifted?
CD: The rapid rate of technological advancement has made it difficult for organizations to stay ahead of new and evolving cybersecurity threats. While businesses are driving their own digital transformations, malicious actors are also leveraging the latest technologies to develop increasingly sophisticated hacking techniques. In particular, the rise in artificial intelligence (AI) and machine learning (ML) enables cybercriminals to initiate attacks with fewer resources or skills, using advanced capabilities such as automated vulnerability scanning.
One key area of concern that has emerged in today's digital landscape is identity and access management. The widespread adoption of cloud services, IoT devices, and third-party integrations, along with remote working, has led to a proliferation of permissions and privileged identities. The more technologies used by an organization, the more identities each user will have -- typically across multiple locations, accounts, and devices.
This means that to breach an entire network of systems and data, cybercriminals only need to gain entry through any one of these user access points by compromising the identity of a single individual. To further complicate matters, many businesses are now operating in a mixed computing environment with disconnected on-premise and cloud applications -- making it even more difficult to manage and secure identity and access.
BN: Why has identity, in particular, become such a challenging vector to protect?
CD: With the increasing complexity and number of identities held by organizations, it is unsurprising that there has also been a surge in attacks related to digital identities. In 2023 alone, a staggering nine out of 10 US businesses reported an identity-related security breach.
But the reality is, software rarely causes data breaches -- people do. Human trust and emotions can be exploited by cybercriminals in a range of ways:
- Social engineering attacks trick individuals into providing sensitive information, login credentials, or access to restricted systems. One example is 'phishing' where the user receives an email containing a link which downloads malware onto their device.
- Password spraying is when a cybercriminal uses default or common passwords against multiple user accounts. This way the attacker can avoid account lockouts that are triggered through multiple login attempts on the same account.
- Credential stuffing involves using stolen login credentials -- usually from a data breach -- to access user accounts on other platforms. This is possible where people use the same password across different accounts.
- Account takeovers occur by gaining control of an account, using techniques like the ones mentioned above. The hacker can then use the account to steal data, make fraudulent transactions, or launch further attacks.
The problem is that human behavior is very difficult to control. For example, despite the cybersecurity risks, over 60 percent of people use the same passwords across multiple websites and platforms. This is why identity and access management is extremely challenging for most organizations. It requires robust procedures and systems to effectively manage identity risks and prevent incidents before they occur.
BN: How can AI help secure identity?
CD: It is becoming clear that traditional security approaches are no longer adequate for identity and access management (IAM). This is where AI-based solutions could hold the solution: by integrating AI and ML into security systems, an organization can significantly enhance their threat detection and response capabilities. That’s because these technologies are able to capture and process large volumes of data to automate and streamline IAM decision-making.
During the authentication process, AI can accurately and efficiently verify the identity of users, analyzing key factors including biometrics, device information, and user behavior. An AI-based IAM automatically assigns privileges and roles to each user, based on the minimum level of access required to perform their job at any given time.
Advanced AI algorithms continuously monitor all identity and access activity to identify suspicious or atypical behavior -- such as unusual login locations, unknown devices, or multiple failed login attempts. These anomalies are instantly flagged to security teams, enabling organizations to respond to cyberthreats in real-time and shut down unauthorized access.
With the collation of vast amounts of data, AI and ML capabilities can also recognize key patterns and trends -- delivering valuable insights to cybersecurity specialists so they can anticipate and prepare for future threats.
BN: What would an example use case look like?
CD: An exciting innovation in the AI space is the integration of knowledge graphs and digital twins into IAM solutions.
Knowledge graphs visualize the relationships between data points, integrating data from various sources across the entire organization. By mapping complex networks of user identities, permissions, roles, and system resources, they create a structured web of interconnected data that AI is able to navigate and analyze for its decision-making. As new roles, rules, and relationships are added to the graph, AI systems can scale and modify IAM dynamically in response to changes.
A digital twin is a virtual replica of an organization's IAM environment, enabling AI to simulate and predict potential changes in identity management -- in order to understand and mitigate their impacts. Because the digital twin is informed by real-time data, it supports ongoing monitoring and predictive analytics, allowing AI to respond swiftly to security threats and prevent breaches before they occur.
When combined, knowledge graphs and digital twin technologies provide a powerful foundation for AI-enabled IAM solutions, underpinned by a dynamically-updated data model -- helping organizations achieve a comprehensive view of their IAM structures.
BN: What is the role of data infrastructure in supporting AI for protecting identity?
CD: When it comes to identity protection, AI is only as effective as the data infrastructure that supports it. This is why foundational technologies -- such as knowledge graphs and digital twins -- play a crucial role in enabling advanced AI capabilities through efficient and accurate data collection, processing and security.
Here are key aspects of how data infrastructure allows AI to detect and prevent identity threats:
- Data integration. Identity data is collected from multiple sources and stored in a centralized repository -- facilitating easy access and integration for AI systems. A robust data infrastructure also ensures that data is accurate, up-to-date, and relevant.
- Data processing. AI systems require large volumes of identity data to learn from and make predictions. Scalable data infrastructures can process very large data sets to enable contextual, informed decision-making.
- Data security. Data infrastructure uses encryption to protect identity data both in transit and at rest. It also implements strict access controls to ensure that data is not exposed to unauthorized access and the risk of data breaches.
- Data governance. Organizations must comply with strict rules and standards related to data privacy and protection. Data infrastructure ensures regulatory compliance, maintaining detailed audit trails to provide transparency and accountability.
- Data analytics. Data infrastructure transforms raw identity data into meaningful insights that AI models can use for training and prediction. It also facilitates testing and validation of AI models to produce the best results.
- Data monitoring. By continuously monitoring data for identity security threats, and automatically informing appropriate sources, data infrastructure ensures the ongoing protection of identity data.
Image credit: Milkos/depositphotos.com